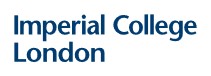
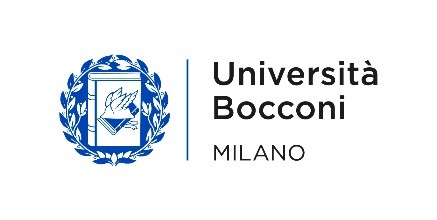
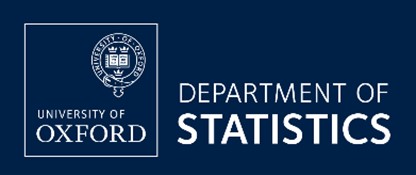
EPSRC StatML CDT Summer School in Causality, Reinforcement Learning and Statistical Learning
Modern Statistics and Statistical Machine Learning programme
Sunday 23rd July to Friday 28th July at Missenden Abbey, Great Missenden, Bucks.
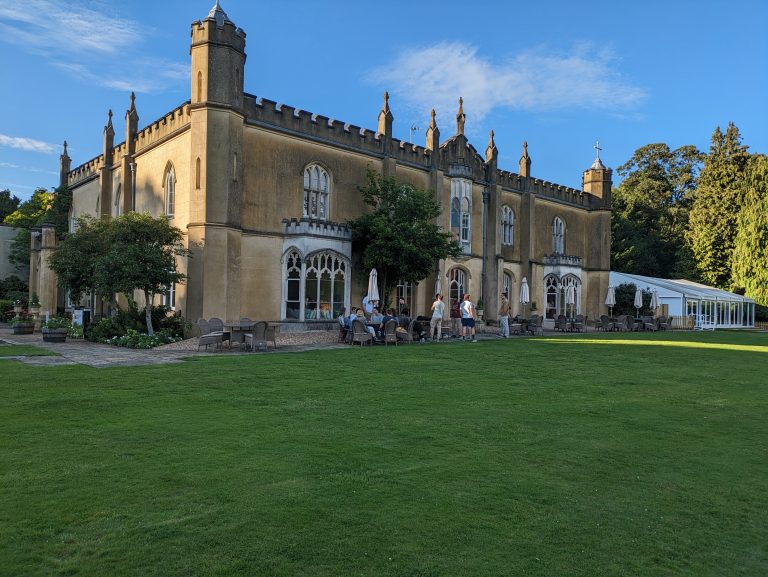
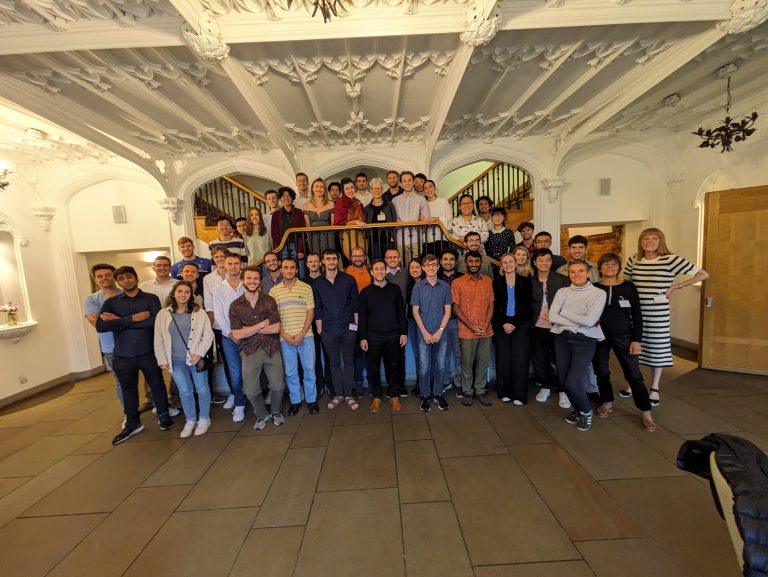
The 2023 Summer School in Modern Statistics and Statistical Machine Learning (StatML) took place at Missenden Abbey in Bucks and was organised by the cohort-based doctoral programme in Modern Statistics and Statistical Machine Learning and Bocconi University. The school featured courses by Susan Murphy (Harvard University) on Causality and Reinforcement Learning and by Alessandro Rudi (INRIA, Paris) on Statistical Learning Theory, as well as presentations by students from both the StatML and Bocconi University.
Our guest lecturers were:
Professor Susan Murphy
Mallinckrodt Professor of Statistics and of Computer Science.
Radcliffe Alumnae Professor at the Radcliffe Institute, Harvard University.
http://people.seas.harvard.edu/~samurphy/
Susan Murphy’s research focuses on improving sequential, individualized, decision making in digital health. She developed the micro-randomized trial for use in constructing digital health interventions; this trial design is in use across a broad range of health-related areas. Her lab works on online learning algorithms for developing personalized digital health interventions. Dr. Murphy is a member of the National Academy of Sciences and of the National Academy of Medicine, both of the US National Academies. In 2013 she was awarded a MacArthur Fellowship for her work on experimental designs to inform sequential decision making. She is a Fellow of the College on Problems in Drug Dependence, Past-President of Institute of Mathematical Statistics, Past-President of the Bernoulli Society and a former editor of the Annals of Statistics.
Dr Alessandro Rudi
Researcher at INRIA and École Normale Supérieure, Paris
Dr Rudi’s main area of research is machine learning, including theory, algorithms and applications at École Normale Supérieure and INRIA, Paris. In 2021 he was awarded of an ERC starting grant with the goal of laying the foundations of a solid theoretical and algorithmic framework for reliable and cost-effective large scale machine learning on modern computational architectures. He is currently exploring with the team he leads a new direction that promises to bring the same benefits in terms of speed and adaptivity to large scale data that we observe in machine learning to other fields of applied mathematics.
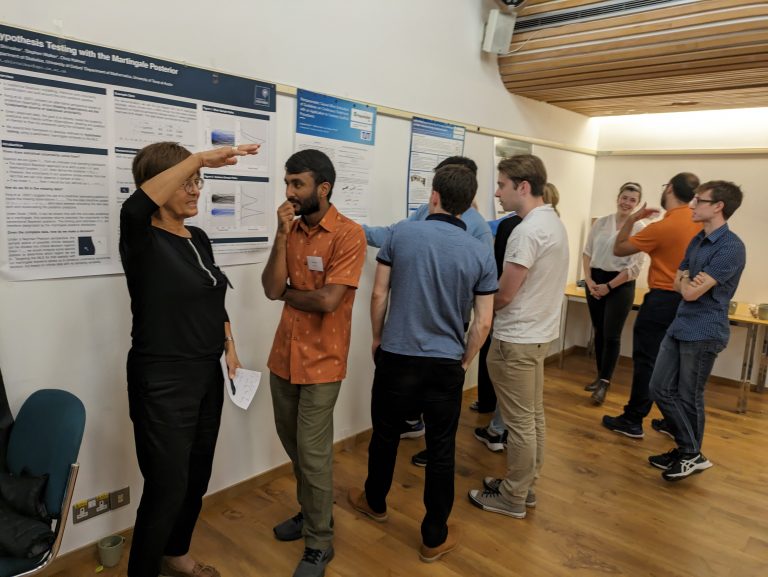