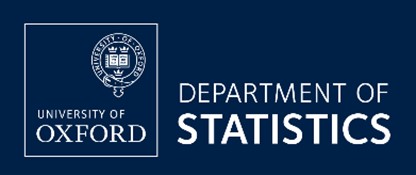
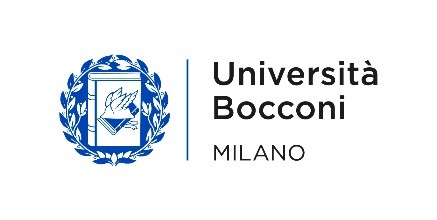

EPSRC StatML and Bocconi Spring School
on Computational Optimal Transport and Statistical Learning with Missing Values
Monday 7th April to Friday 11th April, 2025
with Julie Josse, on Learning with Missing Values and Gabriel Peyré, on Computational Optimal transport
We are happy to announce that StatML and Bocconi University are joining forces once again in 2024-25 to deliver the Spring School on Computational Optimal Transport and Statistical Learning with Missing Values.
The programme will take place at Cumberland Lodge, Windsor, from Monday April 7th to Friday April 11th 2025.
Applying to the Spring School
The StatML and Bocconi Spring School welcomes applications from external students. To be eligible, you must either: 1) Be an active postgraduate student (masters or DPhil/PhD), or 2) a Postdoctoral researcher.
To apply to the Spring School, you will need to fill this form (click here). In addition to this form, you will need to submit a 1) personal statement (max 500 words), 2) your most recent transcript and 3) your resume (CV), to Frédérique Godin at statmlcdt@stats.ox.ac.uk. Please note that you do not need to submit your transcript if you are a PhD/DPhil or a Postdoctoral researcher.
Your application and supporting documents must be submitted by Friday 31st January 2025, midday UK time. In case of questions, please reach out to Frédérique Godin at statmlcdt@stats.ox.ac.uk.
Once submitted, your application will be assessed by our steering committee. Please see the timeline below:
Registration is now open
Registration closes : 31/01/2025
Response sent back on applications: around 10/02/2025
Deadline for payment : 28/02/2025
Due to limited places, it is possible that some applications will be waitlisted. If your application has been waitlisted, you can expect a definitive answer from the Spring School’s committee after February 13, when selected applicants will have confirmed their presence to the course.
Selected participants are responsible for getting to Cumberland lodge. We are currently looking in ways to ease the transport from different locations and options will be shared with the participants closer to the date.
If you have any questions related to the Spring School, please get in touch with Frédérique Godin at statmlcdt@stats.ox.ac.uk
Costs
The cost of the Spring School is £343 per participant. This cost is inclusive of accommodation and all meals for the week.
Course Content and description
Our invited speakers for this 2024-25 edition of the Spring School are Julie Josse, from Inria and Gabriel Peyré, from École Normale Supérieure. Please read below for the course descriptions and speakers bios.
Learning with Missing Values, with Julie Josse
Missing data is a ubiquitous and critical challenge in data analysis. In this series of lectures, we will begin by exploring classical methods for handling missing data, including simple imputation, multiple imputation, and likelihood-based approaches, developed within an inferential framework. The primary focus will be on accurately estimating parameters and their variances in the presence of missing values. Particular attention will be given to powerful imputation techniques based on low-rank approximations, which are suitable for heterogeneous data types, including both quantitative and categorical variables.
We will then shift to recent advancements within a supervised learning framework, highlighting a counterintuitive yet striking result: naive imputation strategies (such as mean imputation) can be optimal, as supervised learning algorithms effectively handle the complexities. This simplicity has significant practical implications. Furthermore, we will discuss how missing value handling can be seamlessly integrated into tree-based models, such as gradient boosted trees, which have demonstrated strong performance, even in challenging scenarios of non-random missingness.
The lectures will include practical demonstrations with notebooks. Finally, we will briefly address how these techniques apply to causal inference when dealing with missing covariate data.
Julie Josse is a senior researcher at Inria (national research center in digital science), leading the PreMeDICaL team (Precision medicine by data integration and causal learning) in collaboration with Inserm (national research center in health). Her expertise lies in handling missing data, causal inference, and developing machine learning techniques to health data. Before joining Inria in 2020, she was a professor at École Polytechnique, where she also led the Master’s program in data science for business, in partnership with HEC Paris. Julie Josse has been a visiting researcher at institutions like Stanford University and Google Brain Paris, focusing on developing advanced statistical methods, particularly for personalized medicine. She is also known for her contributions to reproducible research and her open-source software development, including R packages like FactoMineR and missMDA. Josse’s current work integrates multi-source clinical data to improve decision-making in healthcare, especially for trauma patient management
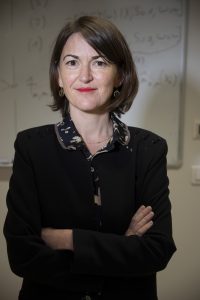
Computational Optimal Transport with Gabriel Peyré
Optimal transport (OT) is a fundamental mathematical theory that intersects optimization, partial differential equations, and probability. It has recently emerged as a crucial tool for addressing a surprisingly wide range of problems in data science, such as shape registration in medical imaging, structured prediction problems in supervised learning, and training of deep generative networks. This mini-course will intertwine descriptions of the mathematical theory with recent developments in scalable numerical solvers.
This approach will underscore the significance of recent advancements in regularized approaches for OT, which make it possible to address high-dimensional learning problems. In the first part of the course, I will explain Monge’s original transportation problem and detail Brenier’s theorem, which guarantees the existence and uniqueness of the optimal map. This theorem is then applied in the contexts of Gaussian distributions and one-dimensional densities. I will then present Kantorovich’s formulation, a convex relaxation that has a strong connection with linear programming.
In particular, I will explain the celebrated Birkhoff-von Neumann theorem, which establishes an equivalence between Monge’s and Kantorovich’s formulations. I will also discuss the metric properties of Optimal Transport, which define a metric capable of quantifying the convergence in law of distributions and making it a powerful loss function in imaging and learning. The last part will focus on high-dimensional problems, requiring the use of entropic regularization. I will draw the connection of this approach with the Schrödinger problem in statistical physics and highlight the associated Sinkhorn’s algorithm, which is highly parallelizable on GPUs and has a significant connection with deep learning and neural networks. Materials for the course, including a small book, slides, and computational resources, can be found online at https://optimaltransport.github.io/.
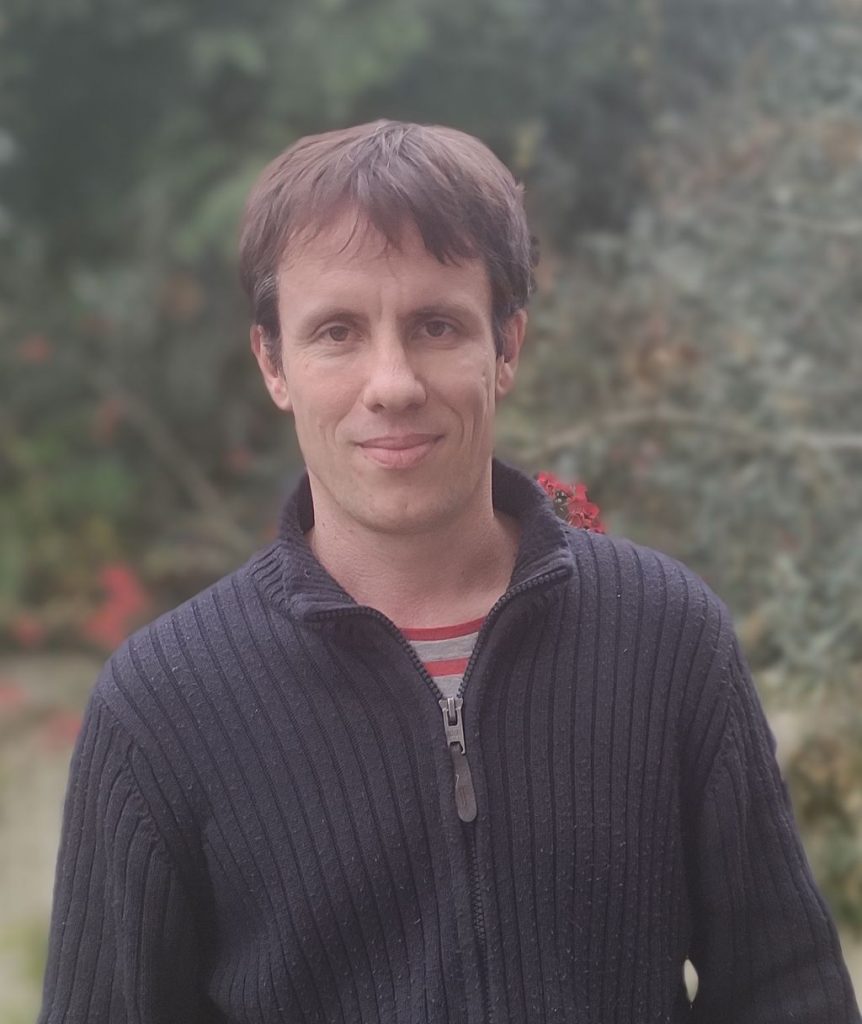